Deep Dive Into Llm Embedding Model Performance What To Look For In A

Deep Dive Into Llm Embedding Model Performance What To Look For In A Leaderboards have a specific purpose, and this video goes in details about the ways in which large language models are compared for their performance. detail. Llm architectures explained: nlp fundamentals (part 1) deep dive into the architecture & building of real world applications leveraging nlp models starting from rnn to the transformers. 5d ago.
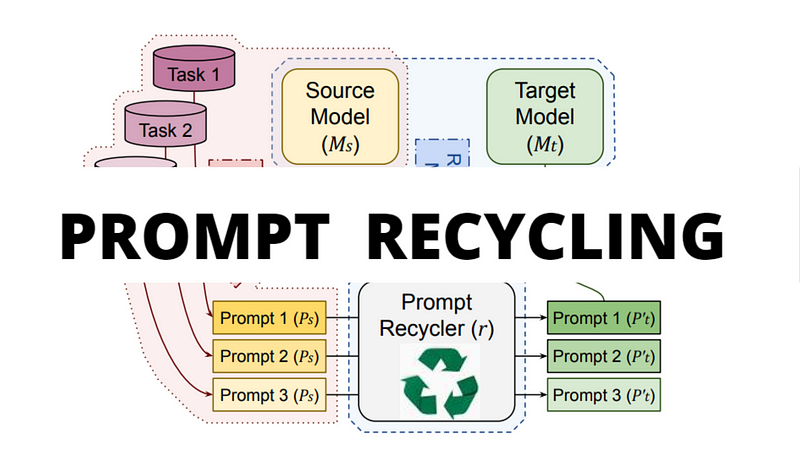
Mastering The Art Of Prompt Engineering A Deep Dive Into Large In our second post, inside look: exploring ollama for on device ai, we took a deep dive into ollama, a standout framework in the local llm space. we guided you through the installation process, highlighting ollama’s intuitive model registry and its ability to integrate models from hugging face seamlessly. Our work studies how to take a graph and translate it into a format that an llm can understand. we also design a benchmark called graphqa to study different approaches on different graph reasoning problems and show how to phrase a graph related problem in a way that enables the llm to solve the graph problem. we show that llm performance on. Benchmarks and evaluation datasets are created to assess the model’s performance on these tasks. interpretability impact: probing tasks help researchers understand what linguistic information is captured by different layers, providing insights into the model’s internal representations. human ai collaboration interfaces:. Conclusion in this blog post, we compared the performance of three large language models (llms) roberta, mistral 7b, and llama 2 for disaster tweet classification using lora.
Letтащs юааdeepюаб юааdiveюаб юааintoюаб An юааembeddingюаб юааmodelюаб In An юааllmюаб Benchmarks and evaluation datasets are created to assess the model’s performance on these tasks. interpretability impact: probing tasks help researchers understand what linguistic information is captured by different layers, providing insights into the model’s internal representations. human ai collaboration interfaces:. Conclusion in this blog post, we compared the performance of three large language models (llms) roberta, mistral 7b, and llama 2 for disaster tweet classification using lora. When training an llm, you are essentially trying to optimize all the mathematical computations that happen in the model with the input embeddings to create the desired output. a deep dive into. 1. two step process: the rag llm operates in a two step process: retrieval: the model first searches for relevant documents or passages from a large dataset. this is done using a dense retrieval mechanism, which employs embeddings to represent both the query and the documents.

Fine Tuning Llm A Deep Dive Into Advanced Techniques For Optimal Model When training an llm, you are essentially trying to optimize all the mathematical computations that happen in the model with the input embeddings to create the desired output. a deep dive into. 1. two step process: the rag llm operates in a two step process: retrieval: the model first searches for relevant documents or passages from a large dataset. this is done using a dense retrieval mechanism, which employs embeddings to represent both the query and the documents.
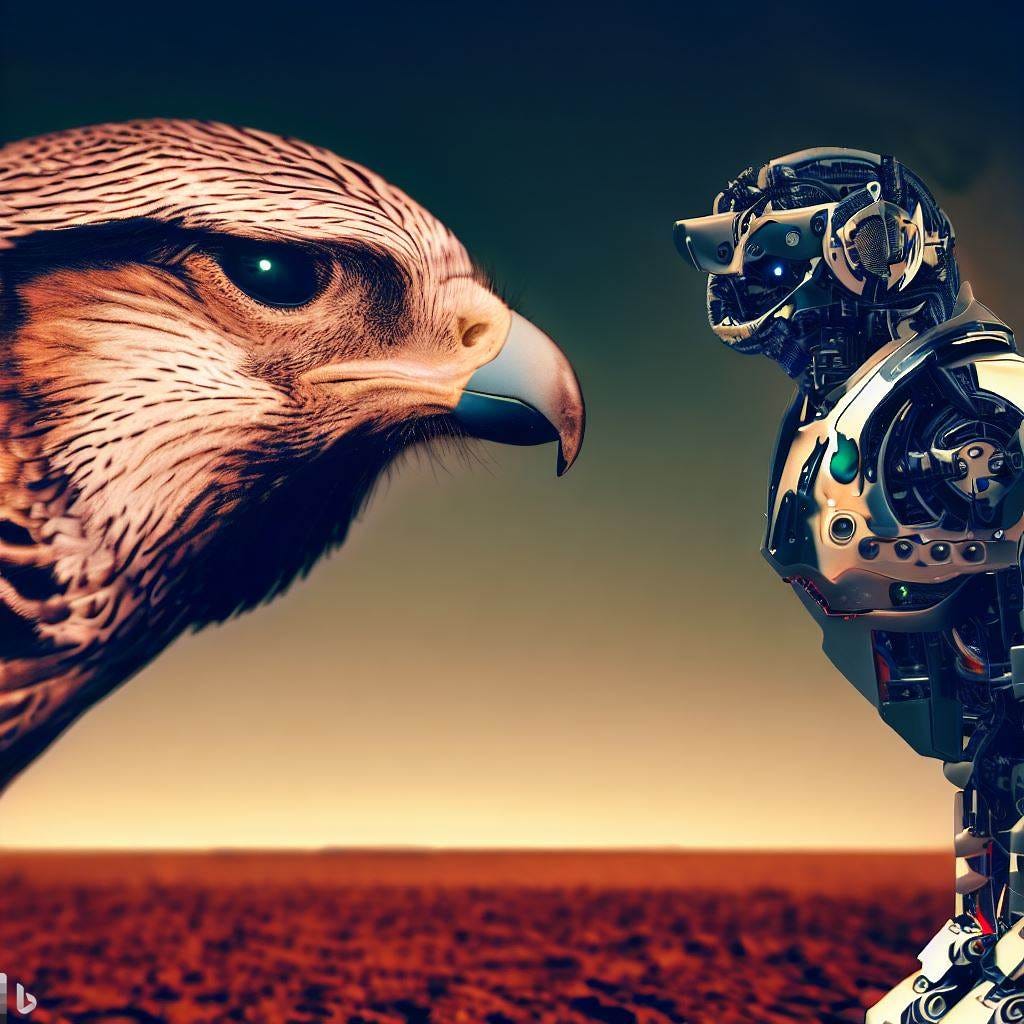
Explaining Falcon 40b Llm A Deep Dive Into Its Performance Training
Comments are closed.