Finetuning Llms Efficiently With Adapters
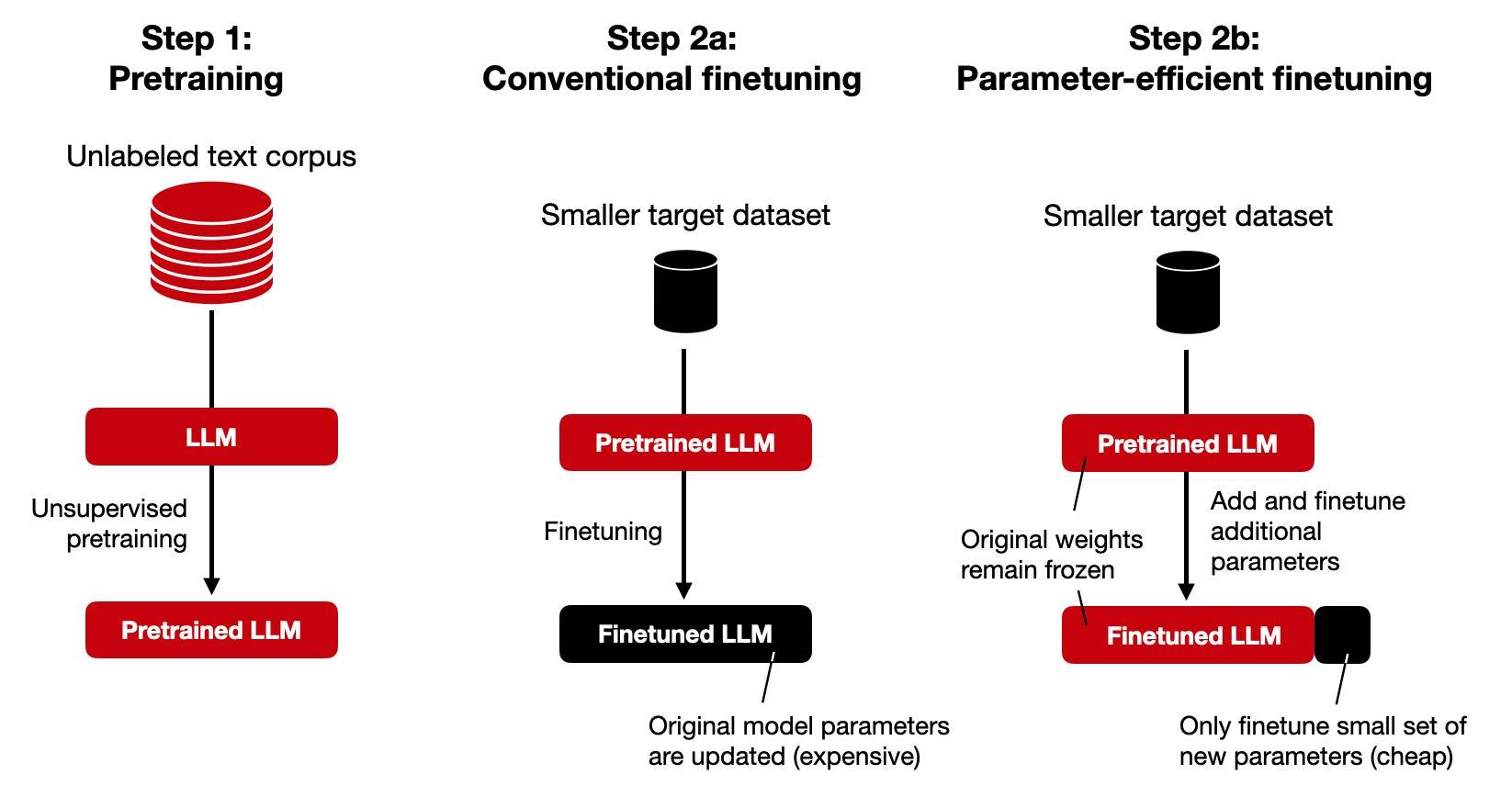
Finetuning Llms Efficiently With Adapters Inserting adapter layers and finetuning all layers. lastly, let's add a control experiment, where we train the model modified with the adapter layers in section 2, but making all parameters trainable. that's 599,424 66,955,010 = 67,554,434 in total. training time: 7.62 min. Lora: 3,506,176. yes, that’s right, full finetuning (updating all layers) requires updating 2000 times more parameters than the adapter v2 or lora methods, while the resulting modeling performance of the latter is equal to (and sometimes even better than) full finetuning, as reported in hu et al. 2021.
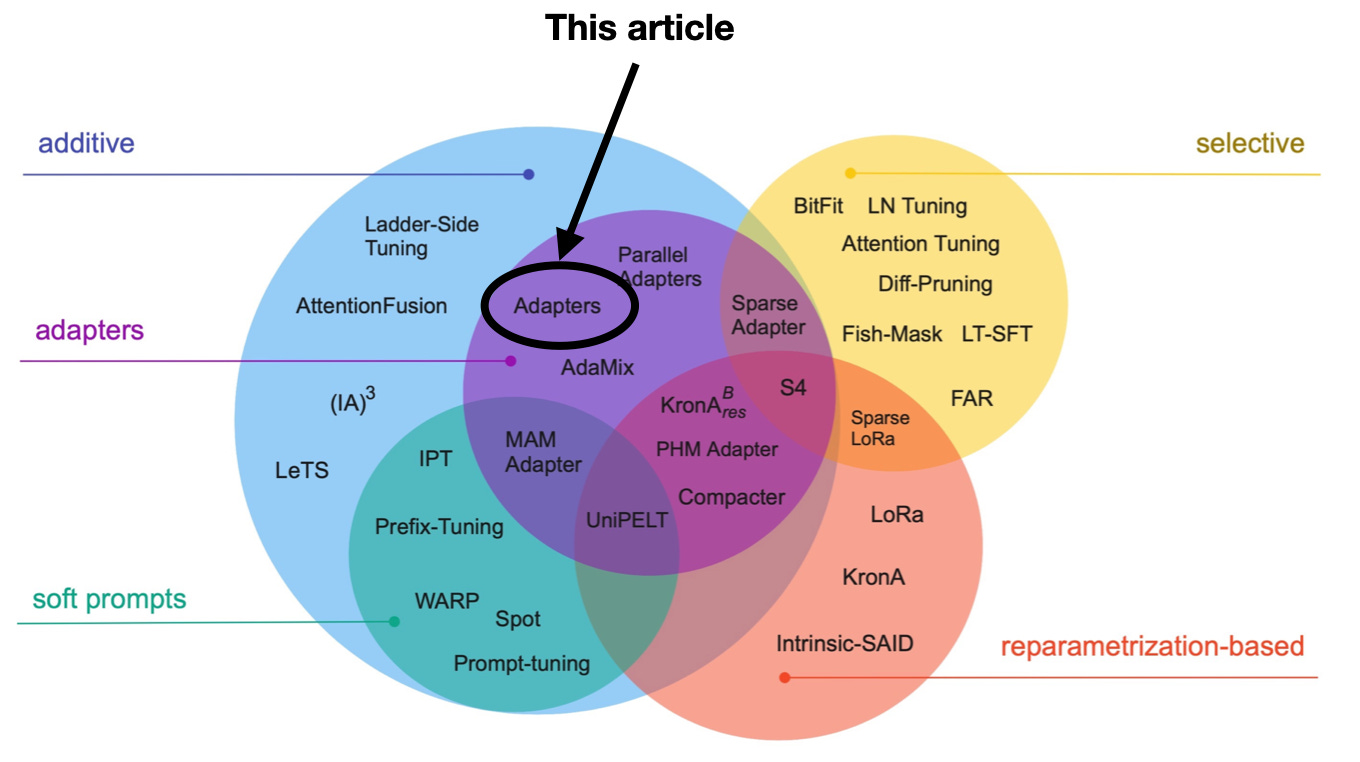
Finetuning Llms Efficiently With Adapters The success of large language models (llms), like gpt 4 and chatgpt, has led to the development of numerous cost effective and accessible alternatives that are created by finetuning open access llms with task specific data (e.g., chatdoctor) or instruction data (e.g., alpaca). among the various fine tuning methods, adapter based parameter efficient fine tuning (peft) is undoubtedly one of the. We present qlora, an efficient finetuning approach that reduces memory usage enough to finetune a 65b parameter model on a single 48gb gpu while preserving full 16 bit finetuning task performance. qlora backpropagates gradients through a frozen, 4 bit quantized pretrained language model into low rank adapters~(lora). our best model family, which we name guanaco, outperforms all previous openly. This approach enhances gpu utilization and reduces kernel launch overhead while maintaining model quality. we have evaluated mlora by fine tuning multiple lora adapters on various publically available llms of different sizes, e.g., tinyllama 1.1b (zhang et al., 2024), llama 2 7b, and 13b (touvron et al., 2023b). %0 conference proceedings %t llm adapters: an adapter family for parameter efficient fine tuning of large language models %a hu, zhiqiang %a wang, lei %a lan, yihuai %a xu, wanyu %a lim, ee peng %a bing, lidong %a xu, xing %a poria, soujanya %a lee, roy %y bouamor, houda %y pino, juan %y bali, kalika %s proceedings of the 2023 conference on empirical methods in natural language processing.
Comments are closed.