Harnessing The Power Of Hugging Face Your Ultimate Guide To Fine
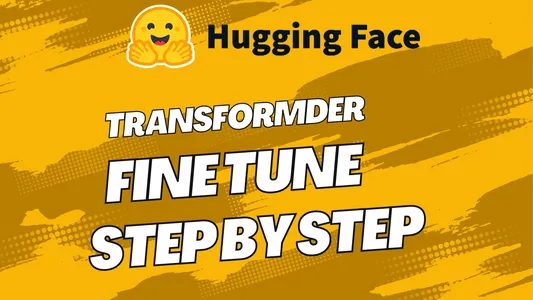
Harnessing Nlp Superpowers A Step By Step Hugging Face Fine Tuning From its simplicity and cost effectiveness to its access to cutting edge models and efficient memory usage, hugging face provides a comprehensive platform for enhancing your nlp projects. let’s delve into some of the key advantages of fine tuning with hugging face: 1. simple and low cost process. fine tuning models with hugging face is a. Join us as we unlock the potential of your data and explore the advanced methodologies that make fine tuning with hugging face an essential practice for any ai enthusiast or professional.

Harnessing The Power Of Hugging Face With Rust A Step By Step Guide Simple fine tuning: the hugging face library contains tools for fine tuning pre trained models on your dataset, saving you time and effort over training a model from scratch. active community: the hugging face library has a vast and active user community, which means you can obtain assistance and support and contribute to the library’s growth. Hugging face: in the ever evolving landscape of artificial intelligence and machine learning, one platform has emerged as a game changer for developers, researchers, and ai enthusiasts alike. Hugging face offers a variety of pre trained models like bert, gpt 2, gpt 3, roberta, and t5, each excelling in different tasks. these models have been trained on vast datasets and can be fine tuned for specific applications, significantly reducing the need for extensive computational resources and time. In this comprehensive guide, we dive deep into sentiment analysis using the state of the art hugging face transformers library. transforming your nlp workflows has never been easier! table of.

Harnessing The Power Of Hugging Face Your Ultimate Guide To Fine Hugging face offers a variety of pre trained models like bert, gpt 2, gpt 3, roberta, and t5, each excelling in different tasks. these models have been trained on vast datasets and can be fine tuned for specific applications, significantly reducing the need for extensive computational resources and time. In this comprehensive guide, we dive deep into sentiment analysis using the state of the art hugging face transformers library. transforming your nlp workflows has never been easier! table of. In this tutorial, we fine tune a roberta model for topic classification using the hugging face transformers and datasets libraries. by the end of this tutorial, you will have a powerful fine tuned model for classifying topics and published it to hugging face 🤗 for people to use. prerequisites. Named entity recognition (ner) in code mixed documents, which have different languages, is hard for natural language processing. in this paper, we use hugging face's multilingual transformers to come up with a way to do code mixed ner without any problems. our work tries to solve the problems that come up when you try to recognise named entities in more than one language within the same text.
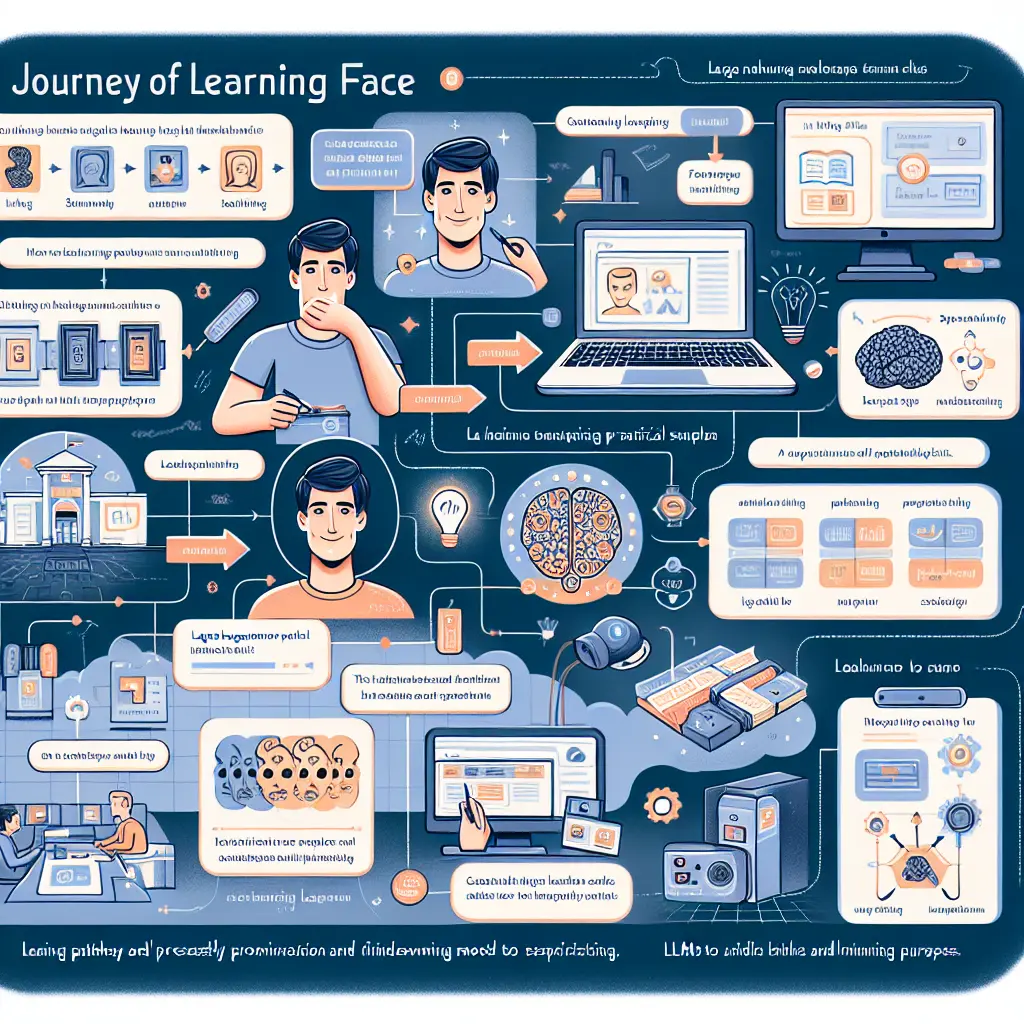
Harnessing Hugging Face For Llm Mastery In this tutorial, we fine tune a roberta model for topic classification using the hugging face transformers and datasets libraries. by the end of this tutorial, you will have a powerful fine tuned model for classifying topics and published it to hugging face 🤗 for people to use. prerequisites. Named entity recognition (ner) in code mixed documents, which have different languages, is hard for natural language processing. in this paper, we use hugging face's multilingual transformers to come up with a way to do code mixed ner without any problems. our work tries to solve the problems that come up when you try to recognise named entities in more than one language within the same text.
Comments are closed.