Insights Applying Machine Learning To Aml Transaction Monitoring
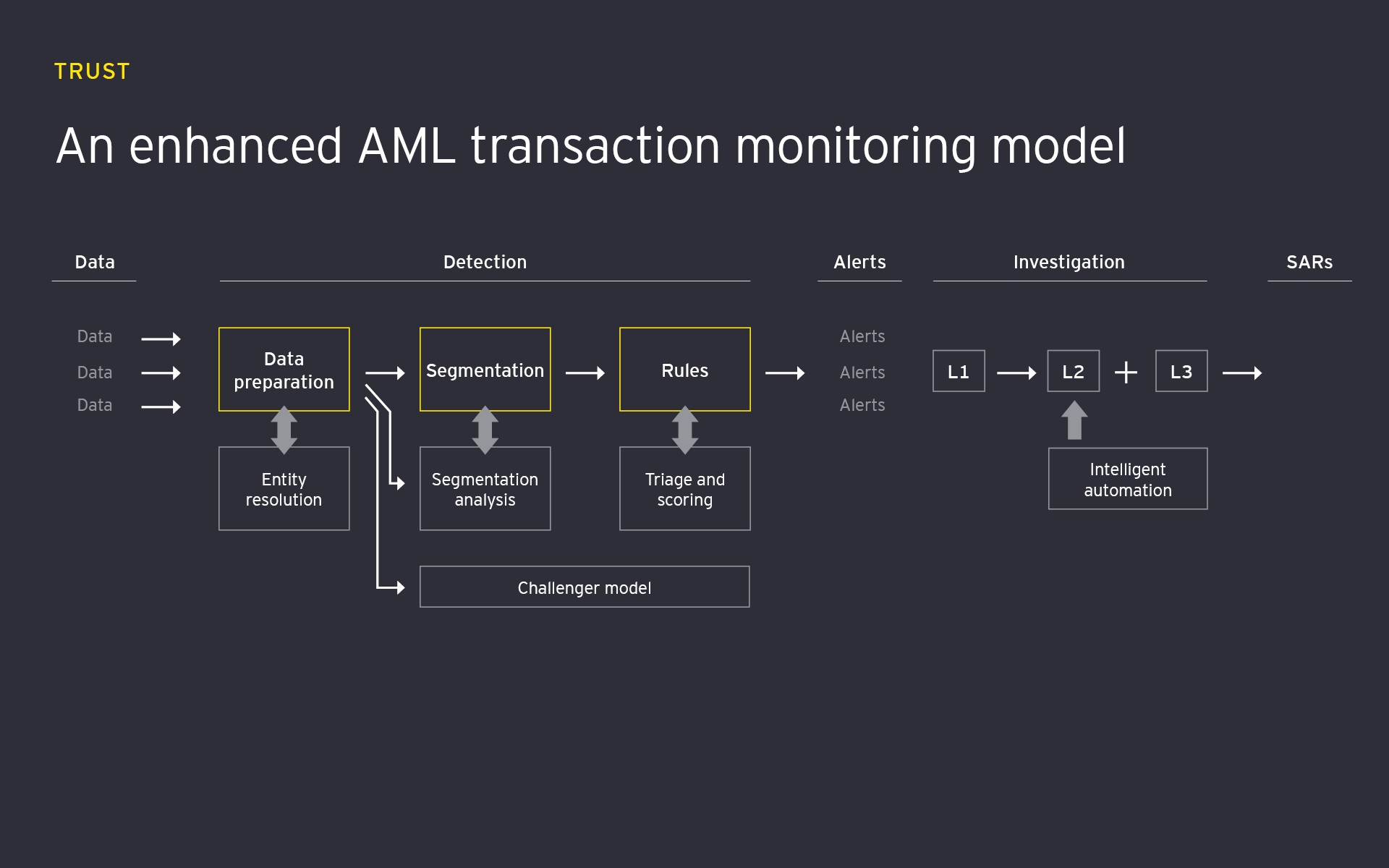
How To Trust The Machine Using Ai To Combat Money Laundering Ey Applying machine learning to transaction monitoring in theory, banks can apply ml across the entire aml value chain (exhibit 1). but we believe that transaction monitoring—specifically, combining ml with other advanced algorithms (for example, random forest, gradient boosting, deep learning)—is where banks can reap one of the most immediate. Machine learning for anti money laundering (ml for aml) 29 august 2023. money laundering is the process of concealing or disguising the sources of illegally obtained money so that it appears to have come from a legitimate source. it is a global threat that has challenged banks for decades. the united nations estimated the cost of global money.

Insights Applying Machine Learning To Aml Transaction Monitoring Youtube In this video based course, kiran kumar shah, head – aml cft, kumari bank nepal provides an insight into concepts such as data science and machine learning,. The integration of ai and machine learning technologies has revolutionized aml transaction monitoring, allowing for more efficient and effective detection of suspicious activities. in this section, we will explore some of the successful applications of ai in aml, including real time data analysis, improved detection of unusual patterns, and. Five benefits of ai driven transaction monitoring systems. 1. adapt to changing behaviors in real time. unlike static rule based systems, ai driven transaction monitoring systems can learn and adjust in real time, staying ahead of emerging risks. machine learning algorithms within these solutions analyze historical transaction data to identify. February 06, 2023. the introduction of machine learning models alongside rules based systems for transaction monitoring can significantly reduce alert volumes while also identifying cases of suspicious activity that would not have been found through the traditional rules based method. this hybrid approach is gaining favour with regulators.
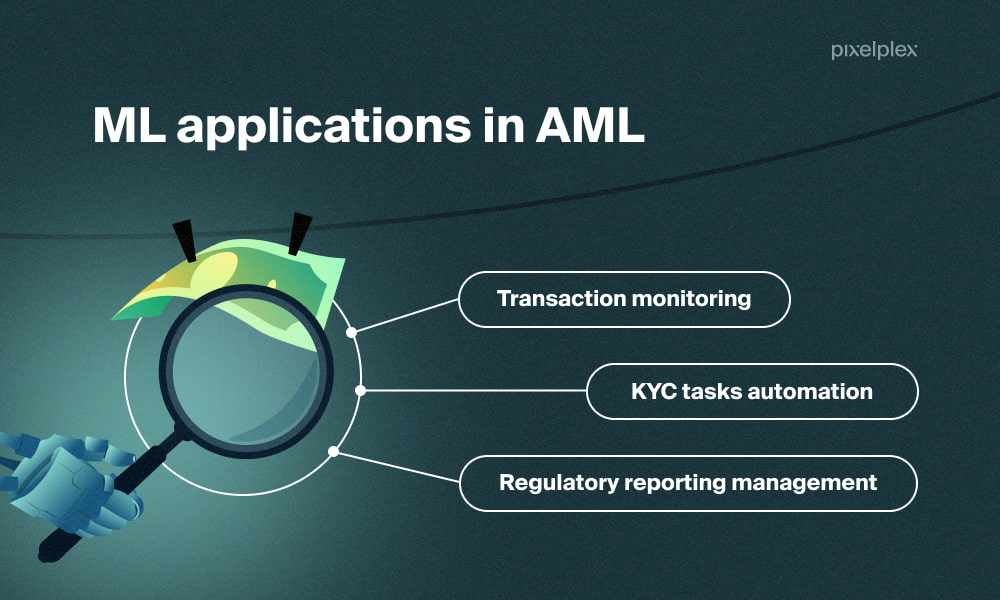
Machine Learning In Aml Benefits Challenges And Applications Five benefits of ai driven transaction monitoring systems. 1. adapt to changing behaviors in real time. unlike static rule based systems, ai driven transaction monitoring systems can learn and adjust in real time, staying ahead of emerging risks. machine learning algorithms within these solutions analyze historical transaction data to identify. February 06, 2023. the introduction of machine learning models alongside rules based systems for transaction monitoring can significantly reduce alert volumes while also identifying cases of suspicious activity that would not have been found through the traditional rules based method. this hybrid approach is gaining favour with regulators. Connecting the insights from transaction monitoring models with customer risk rating models can significantly improve the effectiveness of the latter. while statistically calibrated risk rating models perform better than manually calibrated ones, machine learning and network science can further improve performance. The monitoring of suspicious transactions with respect to money laundering in a typical norwegian bank goes through three stages: the alert stage; the case stage; and the reporting stage (figure 1). these stages also apply to dnb, norway’s largest financial group, from which we have our data.

Machine Learning Cycle For Aml And Tms Using Aml Monitoring Tool To Pr Connecting the insights from transaction monitoring models with customer risk rating models can significantly improve the effectiveness of the latter. while statistically calibrated risk rating models perform better than manually calibrated ones, machine learning and network science can further improve performance. The monitoring of suspicious transactions with respect to money laundering in a typical norwegian bank goes through three stages: the alert stage; the case stage; and the reporting stage (figure 1). these stages also apply to dnb, norway’s largest financial group, from which we have our data.

Deploying Aml Transaction Monitoring Machine Learning Cycle For Aml
Comments are closed.