Lecture 8 Part 1 Derivatives Of Eigenproblems

Lecture 8 Part 1 Derivatives Of Eigenproblems Youtube Mit 18.s096 matrix calculus for machine learning and beyond, iap 2023instructors: alan edelman, steven g. johnsonview the complete course: ocw.mit.ed. Lecture 8 part 1: derivatives of eigenproblems viewing videos requires an internet connection description: to differentiate eigenvectors and eigenvalues, we first consider derivatives with constraints, such as derivatives on a circle or derivatives involving unitary matrices.
Lecture 8 Eigenvalues Eigenvectors And Spectral Theorem Pdf Video. 90 mb. lecture 4 part 2: nonlinear root finding, optimization, and adjoint gradient methods. video. 42 mb. lecture 5 part 1: derivative of matrix determinant and inverse. video. 147 mb. lecture 6 part 1: adjoint differentiation of ode solutions. Lecture 7 part 1: derivatives of random functions lecture 7 part 2: second derivatives, bilinear forms, and hessian matrices lecture 8 part 1: derivatives of eigenproblems. Lecture 8 part 1: derivatives of eigenproblems download 98.5k lecture 8 part 2: automatic differentiation on computational graphs download. Lecture 06 part1: adjoint differentiation of ode solutions: lecture 06 part2: calculus of variations and gradients of functionals: lecture 07 part1: derivatives of random functions: lecture 07 part2: second derivatives, bilinear forms, and hessian matrices: lecture 08 part1: derivatives of eigenproblems: lecture 08 part2: automatic.
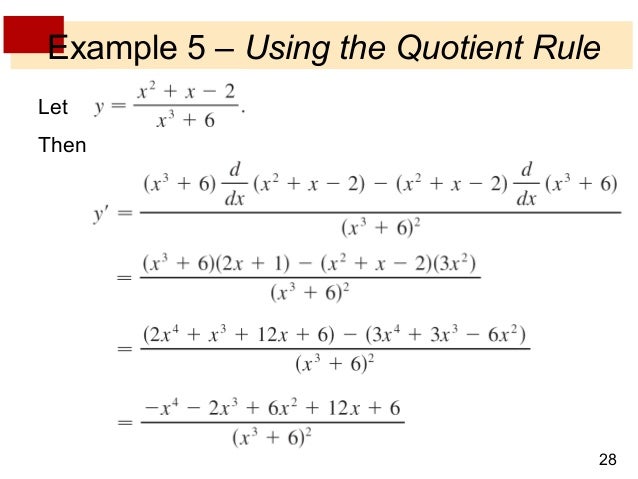
Lecture 8 Derivative Rules Lecture 8 part 1: derivatives of eigenproblems download 98.5k lecture 8 part 2: automatic differentiation on computational graphs download. Lecture 06 part1: adjoint differentiation of ode solutions: lecture 06 part2: calculus of variations and gradients of functionals: lecture 07 part1: derivatives of random functions: lecture 07 part2: second derivatives, bilinear forms, and hessian matrices: lecture 08 part1: derivatives of eigenproblems: lecture 08 part2: automatic. Mit 18.s096 matrix calculus for machine learning and beyond, iap 2023 instructors: alan edelman, steven g. johnson view the complete course: ocw.mit.ed. Part 1: more examples, gradients of scalar valued functions, the chain rule, and forward reverse mode differentiation (slides from lecture 1). course notes: sections 2.3, 3.2, 7.1, 8.3. part 2: matrix jacobians via vectorization; notes: 2×2 matrix jacobians (html) (pluto notebook source code). course notes: sections 4, 5. pset 1, due friday.
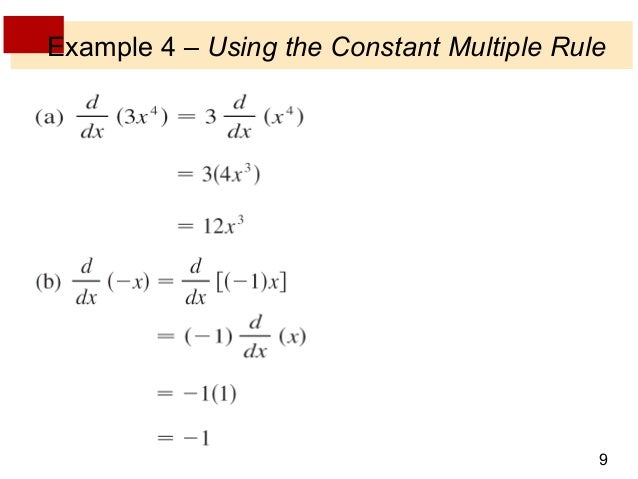
Lecture 8 Derivative Rules Mit 18.s096 matrix calculus for machine learning and beyond, iap 2023 instructors: alan edelman, steven g. johnson view the complete course: ocw.mit.ed. Part 1: more examples, gradients of scalar valued functions, the chain rule, and forward reverse mode differentiation (slides from lecture 1). course notes: sections 2.3, 3.2, 7.1, 8.3. part 2: matrix jacobians via vectorization; notes: 2×2 matrix jacobians (html) (pluto notebook source code). course notes: sections 4, 5. pset 1, due friday.
Comments are closed.