Optimal Transport And Machine Learning 2023
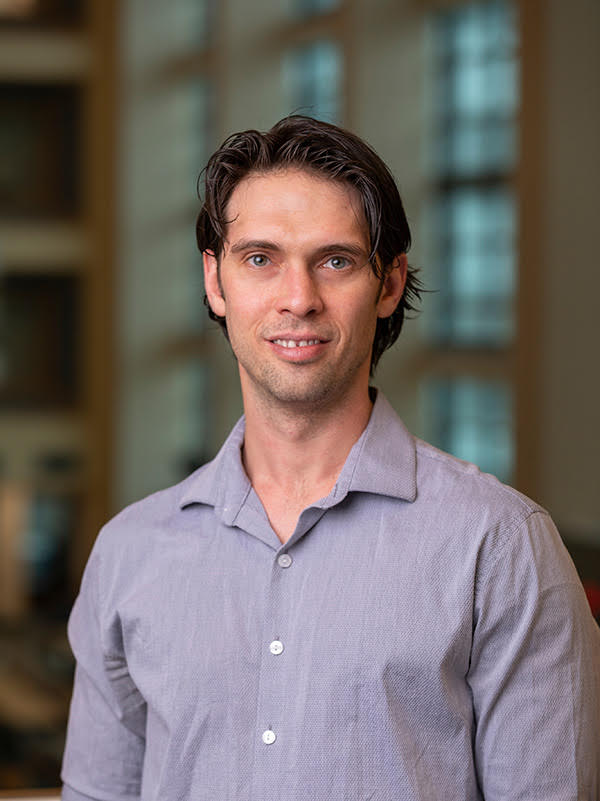
Optimal Transport And Machine Learning 2023 We invite researcher in optimal transport and machine learning to submit their latest works to our workshop. extended deadline for submissions is october 3rd, 2023 aoe. topics include but are not limited to (see call for papers for more details): optimal transport theory. generalizations of optimal transport. Optimal transport and machine learning who received the 2023 abel prize for his seminal contributions to regularity theory for the monge–amp{`e}re equation and.
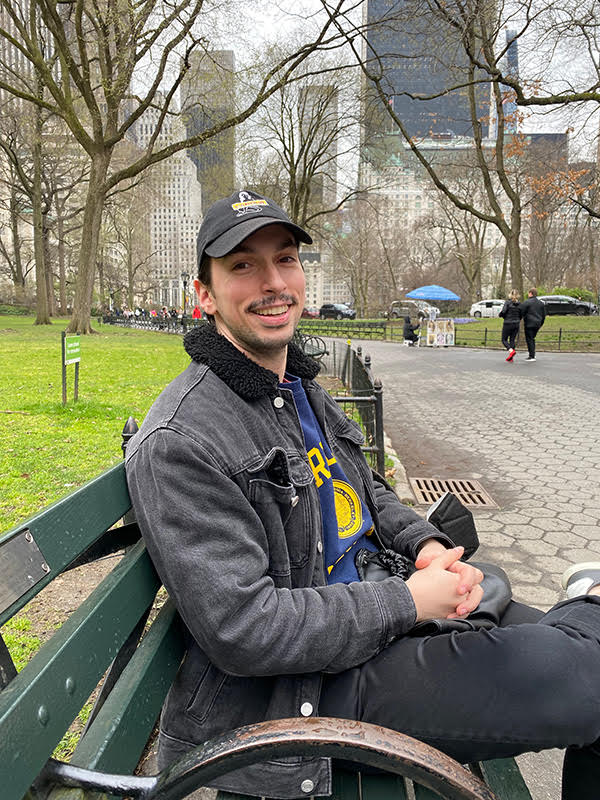
Optimal Transport And Machine Learning 2023 Recently, optimal transport has been proposed as a probabilistic framework in machine learning for comparing and manipulating probability distributions. this is rooted in its rich history and theory, and has offered new solutions to different problems in machine learning, such as generative modeling and transfer learning. in this survey we explore contributions of optimal transport for machine. In this survey we explore contributions of optimal transport for machine learning over the period 2012 – 2023, focusing on four sub fields of machine learning: supervised, unsupervised, transfer and reinforcement learning. we further highlight the recent development in computational optimal transport and its extensions, such as partial. Hyperparameters. to learn the optimal transport maps, we use a batch size of 256, an icnn architecture of 4 hidden layers of width 64, a learning rate of 0.0001 (β1 = 0.5, β2 = 0.9), and λ = 1. Optimal transport (ot) is a mathematical framework that first emerged in the eighteenth century and has led to a plethora of methods for answering many theoretical and applied questions. the last decade has been a witness to the remarkable contributions of this classical optimization problem to machine learning. this paper is about where and how optimal transport is used in machine learning.
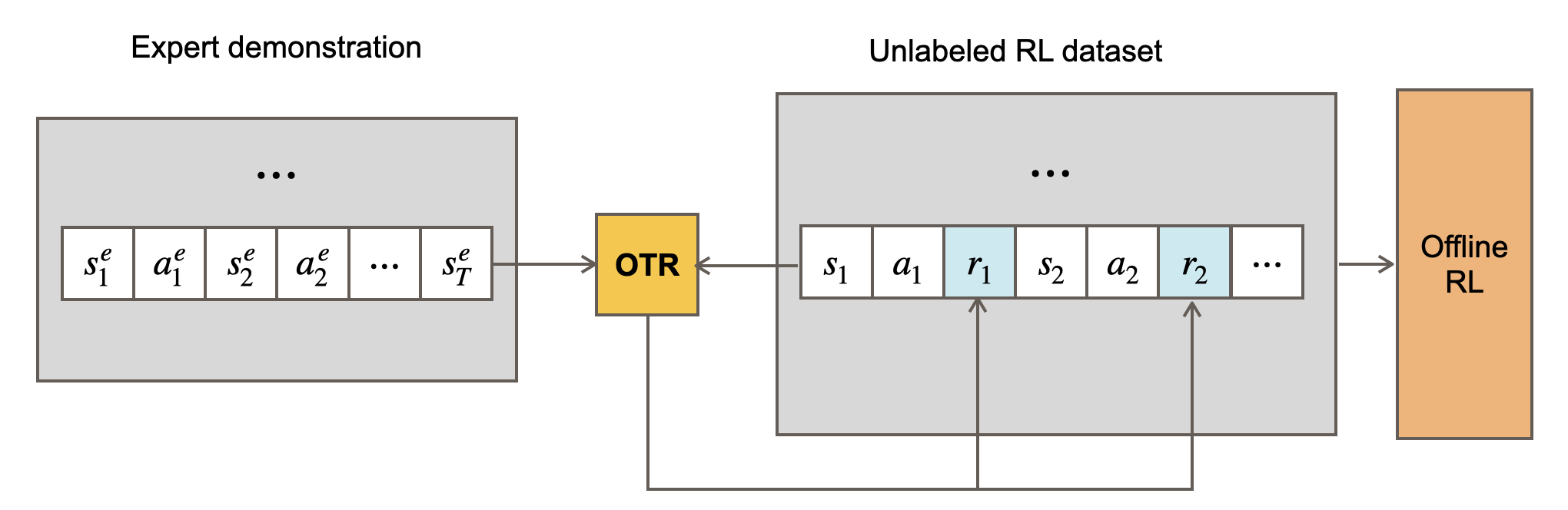
Optimal Transport For Offline Imitation Learning Statistical Machine Hyperparameters. to learn the optimal transport maps, we use a batch size of 256, an icnn architecture of 4 hidden layers of width 64, a learning rate of 0.0001 (β1 = 0.5, β2 = 0.9), and λ = 1. Optimal transport (ot) is a mathematical framework that first emerged in the eighteenth century and has led to a plethora of methods for answering many theoretical and applied questions. the last decade has been a witness to the remarkable contributions of this classical optimization problem to machine learning. this paper is about where and how optimal transport is used in machine learning. Cuturi, marco. "sinkhorn distances: lightspeed computation of optimal transport." advances in neural information processing systems 26 (2013). peyré, gabriel, and marco cuturi. "computational optimal transport: with applications to data science." foundations and trends® in machine learning 11.5 6 (2019): 355 607. The optimal transport and machine learning (otml) workshop series (in '14, '17, '19) has been instrumental in shaping this research thread. for this new installment of otml, we aim even bigger by hosting an exceptional keynote speaker, alessio figalli, who received the 2018 fields medal for his breakthroughs in the analysis of the regularity.
Comments are closed.