Simple Control Utilization Of Demand Forecasting In Smartgrid Figure 2

Simple Control Utilization Of Demand Forecasting In Smartgrid Figure 2 Download scientific diagram | simple control utilization of demand forecasting in smartgrid figure 2. energy generation for ecu microgrid from publication: tuning fuzzy systems to achieve economic. Figure 2 shows the pattern of publications for last two decades within 5 year duration with respect to different time horizons in energy systems forecasting. while ltf stands second in line, most number of publications are made for stf in the period 2016–2021, making it most widely utilized forecasting category in recent times for different applications in grid planning, operations, and.
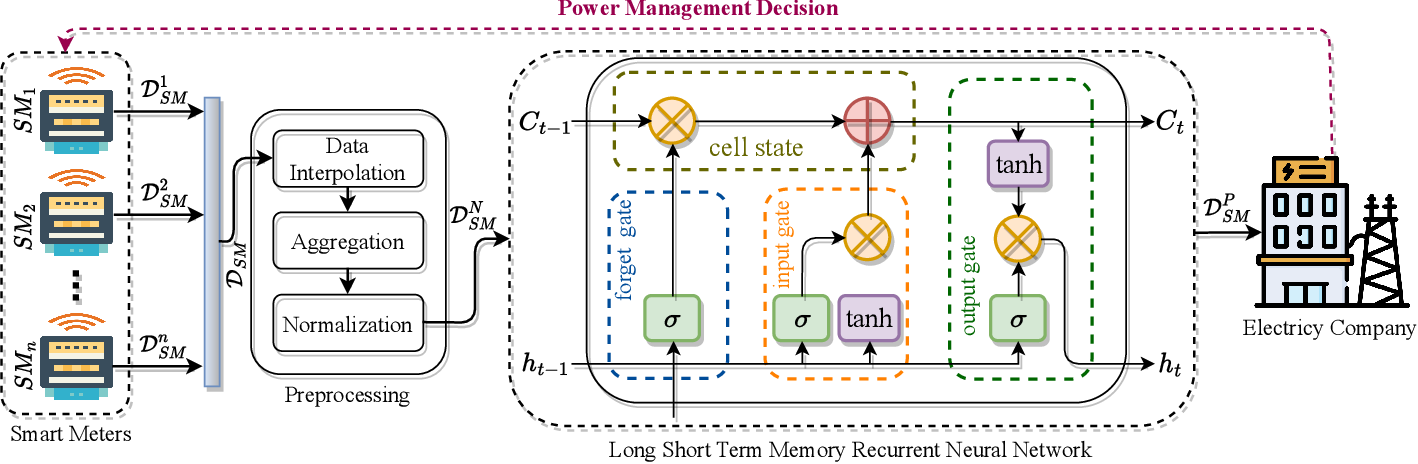
Figure 2 From A Demand And Response Management Model Using Load The new demand pattern is much flatter than the traditional demand pattern in the electricity grid, which reduces the temporal impact on the intraday demand forecast profile in the smart grid. however, factors such as seasonal differences, climate changes, weekends and holidays would still impact the value of demand. 3. The integration of lightgbm demonstrates efficacy in managing the intricate and multidimensional characteristics of smart grid statistics, leading to enhanced accuracy in load forecasting. the research examines the impacts of the hyperparameter tuning with lightgbm and identifies the most effective configurations to improve performance. The ffo algorithm is a population based approach used in smart grid control to address challenges like load balancing, demand response, renewable energy integ ration, and power distribution. Demand response management (drm) plays a crucial role in the prospective development of smart grids. the precise estimation of electricity demand for individual houses is vital for optimizing the operation and planning of the power system. accurate forecasting of the required components holds significance as it can substantially impact the final cost, mitigate risks, and support informed.

Applied Sciences Free Full Text Demand Response Control In Smart Grids The ffo algorithm is a population based approach used in smart grid control to address challenges like load balancing, demand response, renewable energy integ ration, and power distribution. Demand response management (drm) plays a crucial role in the prospective development of smart grids. the precise estimation of electricity demand for individual houses is vital for optimizing the operation and planning of the power system. accurate forecasting of the required components holds significance as it can substantially impact the final cost, mitigate risks, and support informed. Load forecasting: it is an essential function in smart grid that involves predicting the future electricity demand to ensure that the power supply meets the demand . ai techniques such as linear regression, fuzzy neuro model, ann and long short term memory (lstm) can be used to analyze historical data and predict future electricity demand. This study is to review three common artificial intelligence load forecasting methods, including long short term memory, group method of data handling, and adaptive neuro fuzzy inference system that have been used to forecast load in a smart grid consisting of a photovoltaic, wind turbine, battery energy storage system, and electric vehicle.

The Structure Of A Smart Grid Download Scientific Diagram Load forecasting: it is an essential function in smart grid that involves predicting the future electricity demand to ensure that the power supply meets the demand . ai techniques such as linear regression, fuzzy neuro model, ann and long short term memory (lstm) can be used to analyze historical data and predict future electricity demand. This study is to review three common artificial intelligence load forecasting methods, including long short term memory, group method of data handling, and adaptive neuro fuzzy inference system that have been used to forecast load in a smart grid consisting of a photovoltaic, wind turbine, battery energy storage system, and electric vehicle.
Comments are closed.