Stepwise Method Of Multiple Regression Javatpoint
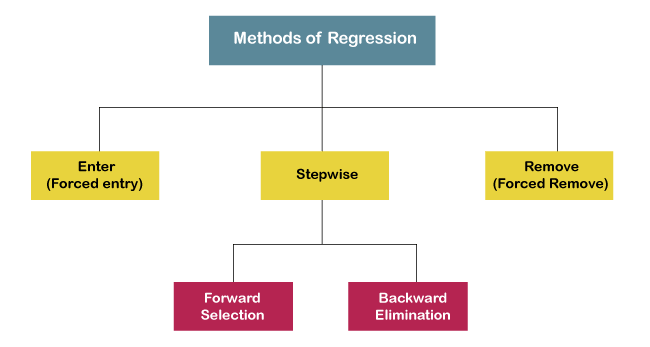
Stepwise Method Of Multiple Regression Javatpoint The first method of regression is the enter method. it is also known as the forced entry method because all the variables are forcefully entered in the model without discrimination on the base of their relative importance. the second method that we have can be called as the stepwise method. this stepwise method of regression contains two methods. The stepwise method of regression analysis is a method in which variables are entered in a model in the format of stepwise criteria. in the model, to enter the variables in a stepwise manner, we have two more methods listed, which are forward and backward methods. forward and backward methods are part of the stepwise regression method. the.
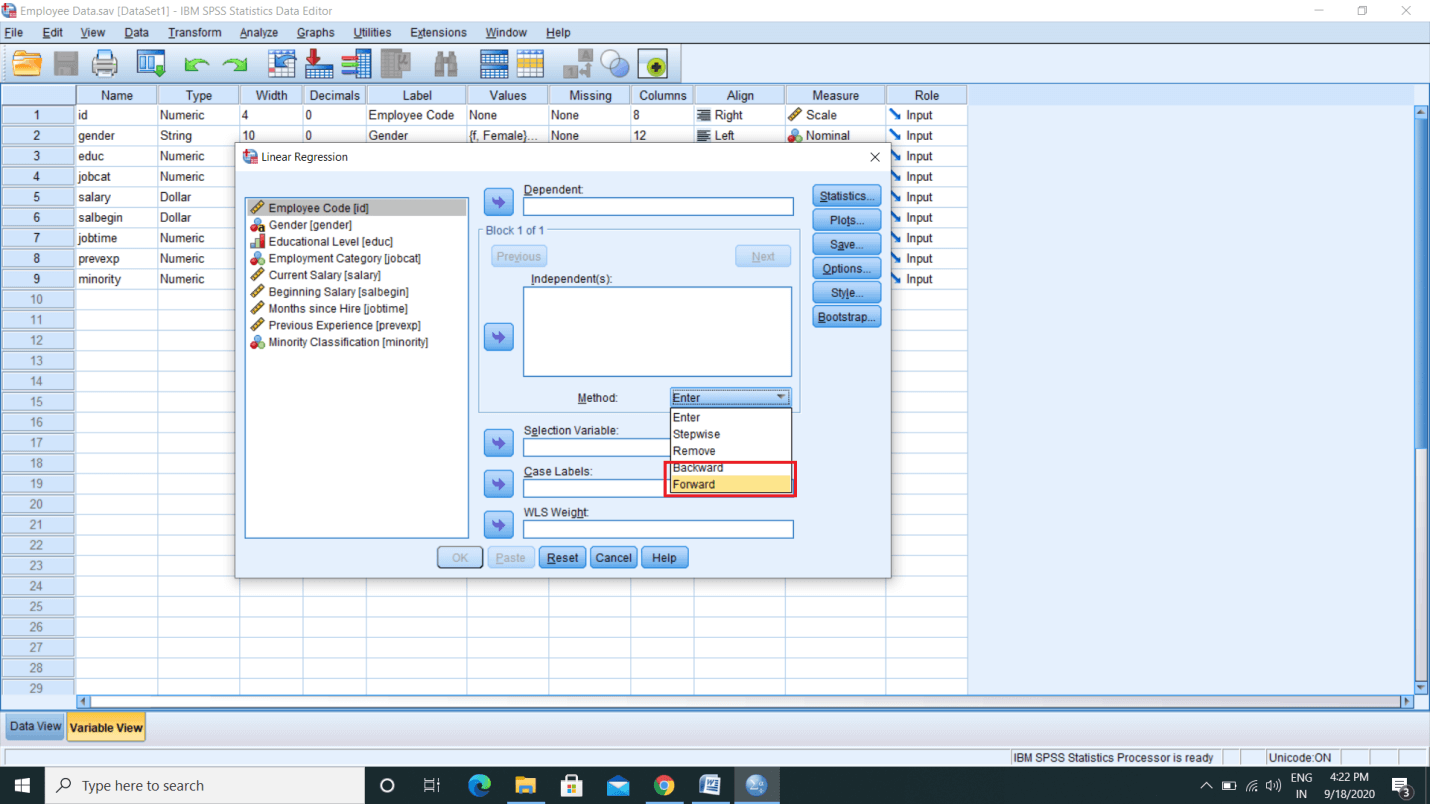
Stepwise Method Of Multiple Regression Javatpoint Multiple linear regression is one of the important regression algorithms which models the linear relationship between a single dependent continuous variable and more than one independent variable. example: prediction of co 2 emission based on engine size and number of cylinders in a car. some key points about mlr:. Regression analysis is a statistical method to model the relationship between a dependent (target) and independent (predictor) variables with one or more independent variables. more specifically, regression analysis helps us to understand how the value of the dependent variable is changing corresponding to an independent variable when other. Stepwise regression is a technique for feature selection in multiple linear regression. there are three types of stepwise regression: backward elimination, forward selection, and bidirectional. One of the most popular techniques for feature selection is stepwise regression. feature selection is the process of selecting a subset of relevant features from the original set of features to improve model performance. in essence, it is about identifying the most informative features that can help the model make accurate predictions.
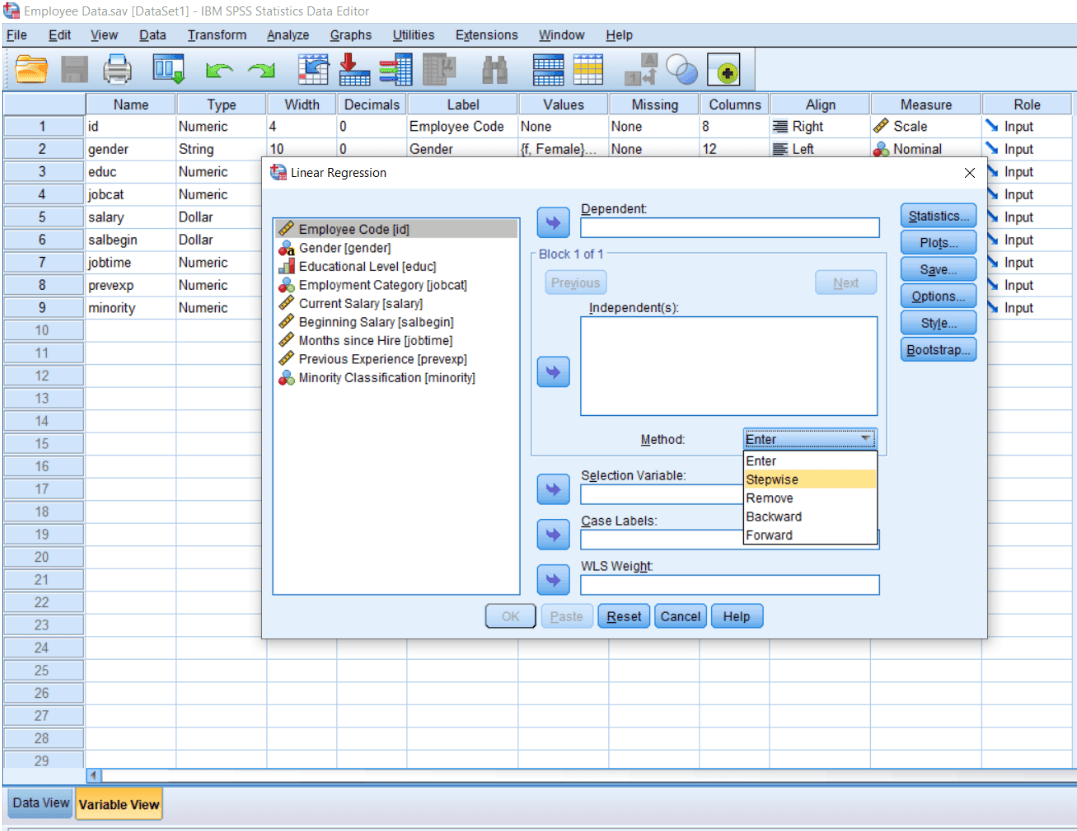
Stepwise Method Of Multiple Regression Javatpoint Stepwise regression is a technique for feature selection in multiple linear regression. there are three types of stepwise regression: backward elimination, forward selection, and bidirectional. One of the most popular techniques for feature selection is stepwise regression. feature selection is the process of selecting a subset of relevant features from the original set of features to improve model performance. in essence, it is about identifying the most informative features that can help the model make accurate predictions. Regression analysis is often called "ordinary least squares" (ols) analysis because the method of determining which line best "fits" the data is to minimize the sum of the squared residuals or erros of a line put through the data. figure 13.8. estimated equation: c = b0 b1lncome e c = b 0 b 1 lncome e. Regression in machine learning. it is a supervised machine learning technique, used to predict the value of the dependent variable for new, unseen data. it models the relationship between the input features and the target variable, allowing for the estimation or prediction of numerical values. regression analysis problem works with if output.
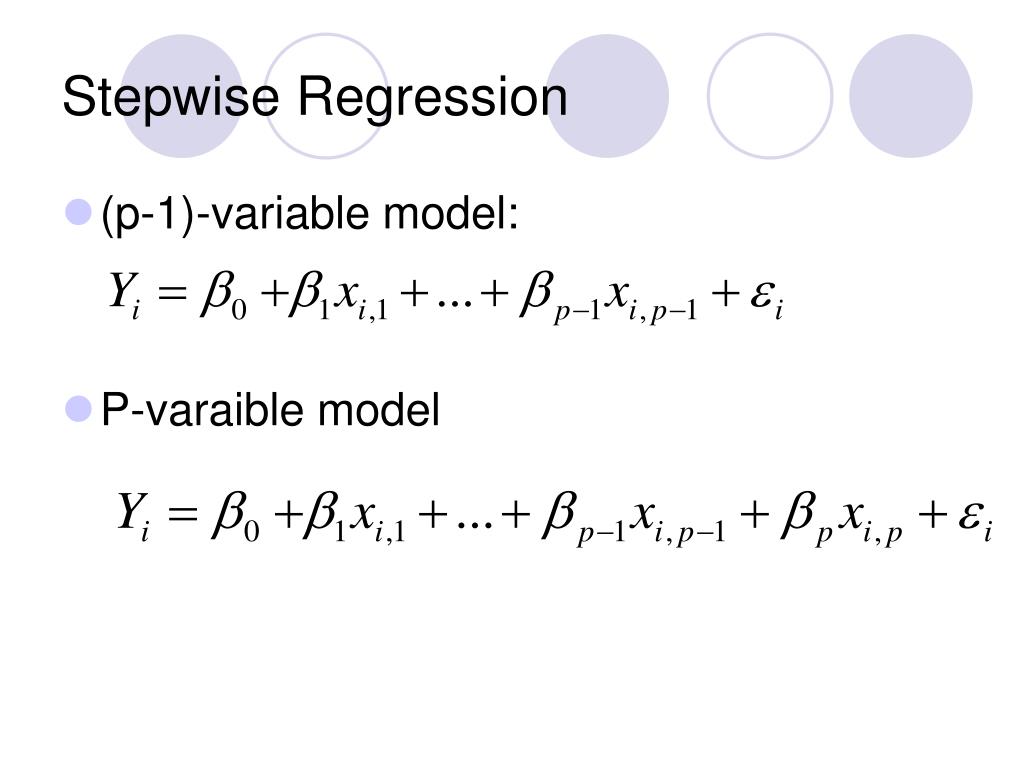
Ppt Chapter 11 Multiple Linear Regression Powerpoint Presentation Regression analysis is often called "ordinary least squares" (ols) analysis because the method of determining which line best "fits" the data is to minimize the sum of the squared residuals or erros of a line put through the data. figure 13.8. estimated equation: c = b0 b1lncome e c = b 0 b 1 lncome e. Regression in machine learning. it is a supervised machine learning technique, used to predict the value of the dependent variable for new, unseen data. it models the relationship between the input features and the target variable, allowing for the estimation or prediction of numerical values. regression analysis problem works with if output.
Comments are closed.